
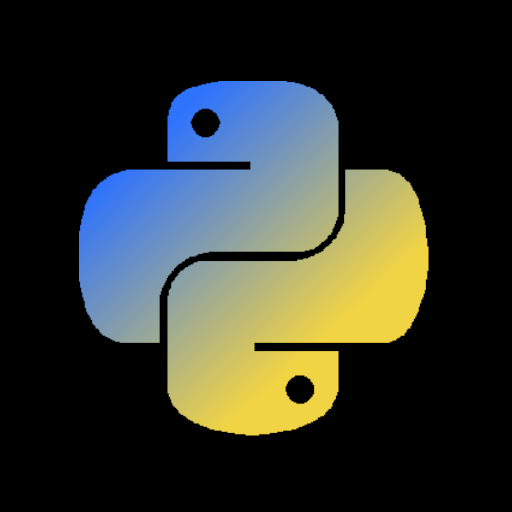
People use Python a lot as a Matlab, Excel/VBA, or R alternative. That was my use for many years. Some of these are compute focused problems and if the dataset is large enough and the computations complex enough then speed can be an issue.
As far as loading packages and printing. Who cares. These are not computationally intensive and are typically IO bound.
I think it depends on how good your Numpy build is. Lot of Numpys are not that well built so Numba seems to help there too in that case.
For a python library to be fast it needs to be compiled for your specific hardware, vectorized, with fast math, and auto parallel. Most are probably not unless you build them youself.